Toward robust and optimal cosmology from galaxy clustering
Extracting cosmological information from galaxy surveys is a difficult task – one to which MPA researchers are now one step closer. Using a theoretical framework known as effective field theory combined with a novel statistical approach, they were able to correctly recover the input cosmology based on a catalog of simplified simulated galaxies.
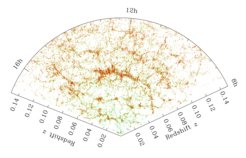
Large galaxy surveys are an extremely important tool for cosmologists, as they allow us to construct a map of the galaxy distribution over a significant fraction of the observable universe (Fig. 1). Such a map contains information on the expansion of the universe, dark energy, dark matter, as well as the early universe. This is the main motivation behind major efforts such as the recently completed Sloan Digital Sky Survey (SDSS) and the European satellite experiment Euclid that is currently under construction. MPA members are participating in both surveys.
The major challenge in interpreting these maps of the universe is that we need a robust model connecting the galaxies to the underlying matter distribution (mostly dark matter). Both theoretical and numerical calculations allow for a reliable prediction for the matter distribution. Galaxy formation is an extremely complex process that we do not understand in detail - and cannot fully simulate either. So how can we hope to extract reliable information about the underlying matter density field and the history of the universe using these objects?
Recently, researchers at MPA have made significant progress by deriving a prediction for the statistical relation between matter and galaxies within a theoretical framework known as effective field theory. This prediction, technically a conditional likelihood, is based on the fact that gravity (as described by Einstein's General Relativity) is the only relevant force on the extremely large scales on which galaxy clustering is measured. Galaxies are relatively small objects when compared to those scales.
The price paid for this rigorous prediction is that it introduces a set of free parameters that capture the details of galaxy formation, and which we cannot predict. These "nuisance" parameters need to be determined from galaxy data, similar to how we estimate the cosmological parameters of interest, such as those that describe the expansion rate of the universe and the properties of dark energy. Fortunately, there is a well-defined set of these free parameters, and the data are expected to be rich enough to constrain both galaxy and cosmological properties at the same time.
The MPA team, along with European colleagues, are now working on taking this approach closer to data. This work is done in the context of the Aquila consortium. The basic idea is to randomly generate likely matter distributions in the observable universe, which we know how to do based on theory, and compare those with the observed galaxy distribution using the statistical relation derived in the effective field theory. This is done using a statistical approach known as Bayesian inference. There are many, many possible matter distributions that could fit our universe, so this is very challenging. Advanced statistical and numerical techniques, however, allow this full Bayesian inference to be feasible even for data sets as big as the SDSS galaxy survey.
![Fig. 2: Ratio of the inferred cosmological parameter (amplitude of matter density fluctuations) to the true value, based on simulated data. A value of 1 corresponds to perfectly accurate inference. The x axis shows the range of scales included in the analysis, with small values corresponding to large scales. The theory is expected to approach the correct answer on large scales (small x value), which is shown to be the case. The blue points show a second-order theoretical prediction, while the red points show the more accurate third-order calculation. The green point uses a more accurate theoretical prediction for the matter density. (From [2]).](/832882/original-1589806246.jpg?t=eyJ3aWR0aCI6MjQ2LCJvYmpfaWQiOjgzMjg4Mn0%3D--76503ec1b606f96e4c3983dd3dfd8282d936d76f)
Fig. 2: Ratio of the inferred cosmological parameter (amplitude of matter density fluctuations) to the true value, based on simulated data. A value of 1 corresponds to perfectly accurate inference. The x axis shows the range of scales included in the analysis, with small values corresponding to large scales. The theory is expected to approach the correct answer on large scales (small x value), which is shown to be the case. The blue points show a second-order theoretical prediction, while the red points show the more accurate third-order calculation. The green point uses a more accurate theoretical prediction for the matter density. (From [2]).
Recently, the MPA team obtained very promising results in a simplified setup. A "galaxy" catalog was constructed from a simulation with known cosmological parameters and matter distribution. The team was able to successfully recover the correct input cosmology to within a few percent (Fig. 2; the cosmological parameter considered here corresponds to the overall amplitude of fluctuations in the matter density). Moreover, this simplified test shows that we understand where the residual error comes from and how it can be improved. The theoretical calculation can be continued to higher orders, and corresponding further improvements are expected.
The next steps are clear: verify that other cosmological parameters are recovered successfully as well, and prepare this approach for the application to real data from large galaxy surveys. This requires much additional work in order to correctly include the complexities of real observational data. The potential gains are tremendous, however: once the Bayesian inference coupled with the likelihood derived from theory does work, we are sure to obtain optimal, robust cosmological constraints from galaxy clustering.